Why Do Data Scientists Struggle Behind the Scenes?
Although data science is an attractive field to work in and is in high demand, similar to other jobs, it has its own challenges. Getting to know which struggles you may face during your data science journey helps you to make wise decisions and not to get disappointed when encountering them. In the following, some of these challenges will be introduced:
Understanding the problem
Some newbie data scientists may think that data science is just doing methods and techniques one after another mechanically; however, a proper understanding of the problem and asking appropriate questions is an essential key that can be easily forgotten. This challenge can be solved over time when you get used to communicating with business stakeholders, understanding data science objectives within your company, and getting to know the features of the data you will deal with.
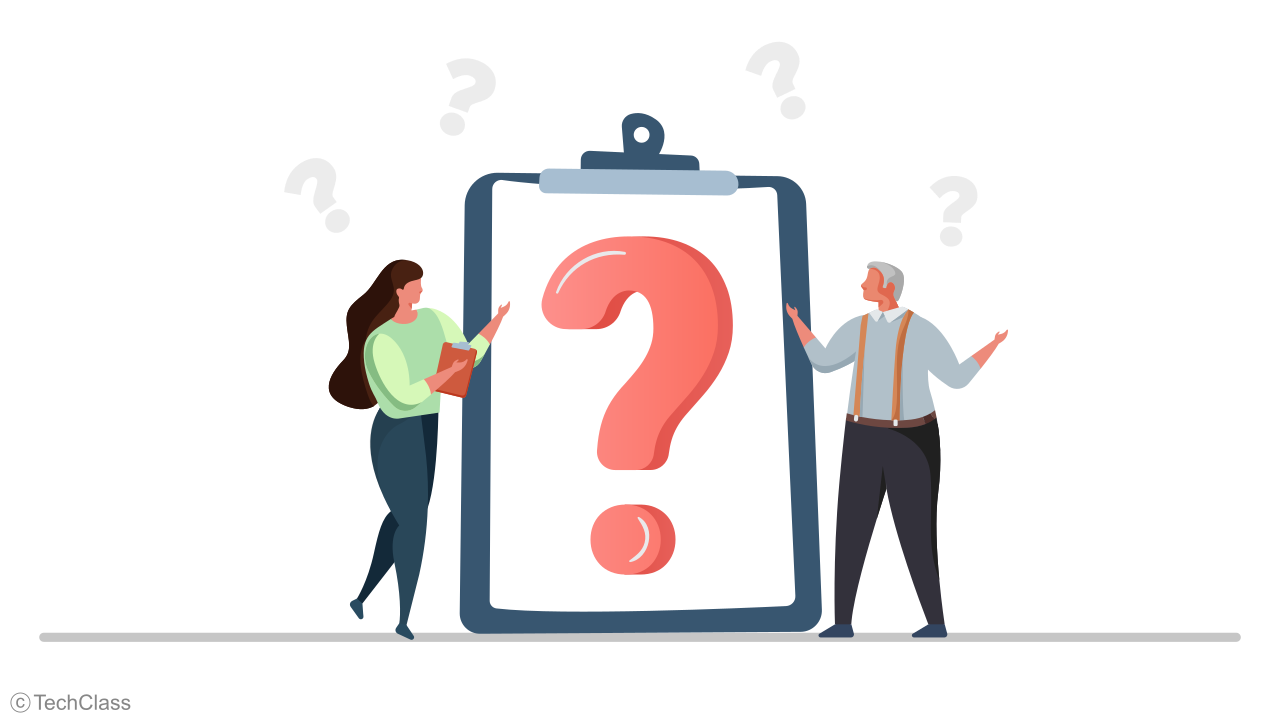
Here's why understanding the problem is essential:
- Guides the entire project: By clearly comprehending the problem, you can tailor your approach, decide on the right methods to employ, and ensure that the results are relevant and actionable.
- Facilitates effective communication: When you grasp the intricacies of the problem, you can communicate more efficiently with stakeholders, ensuring that everyone is on the same page. This reduces misunderstandings and helps to align objectives.
- Informs data collection and preparation: A clear problem statement will guide you on what data is relevant, how to clean it, and how to prepare it for analysis. Without this understanding, you risk wasting time on irrelevant data or overlooking crucial information.
- Enhances model selection and evaluation: Different problems might require different approaches. Knowing the problem inside and out helps in choosing the most suitable model and evaluating its performance in a meaningful way.
Handling messy data
Some may think that data science is just using advanced models and tools. However, you should know that real-world data is messy before any modeling. Most of the time, a data scientist is dedicated to handling raw data and preparing it in the right format for the next steps. This seems somehow frustrating to some people who have started to work in this field.
Understanding the nature of real-world data is crucial. Data streams from a myriad of sources, whether it's user inputs, sensors, or digital transactions. Along the way, it picks up noise. This "noise" manifests as missing values, duplicates, and inconsistencies. Reading raw data is like reading a book with missing pages, repeated sections, and confusing sentences. To understand the story, you need to fix these problems.
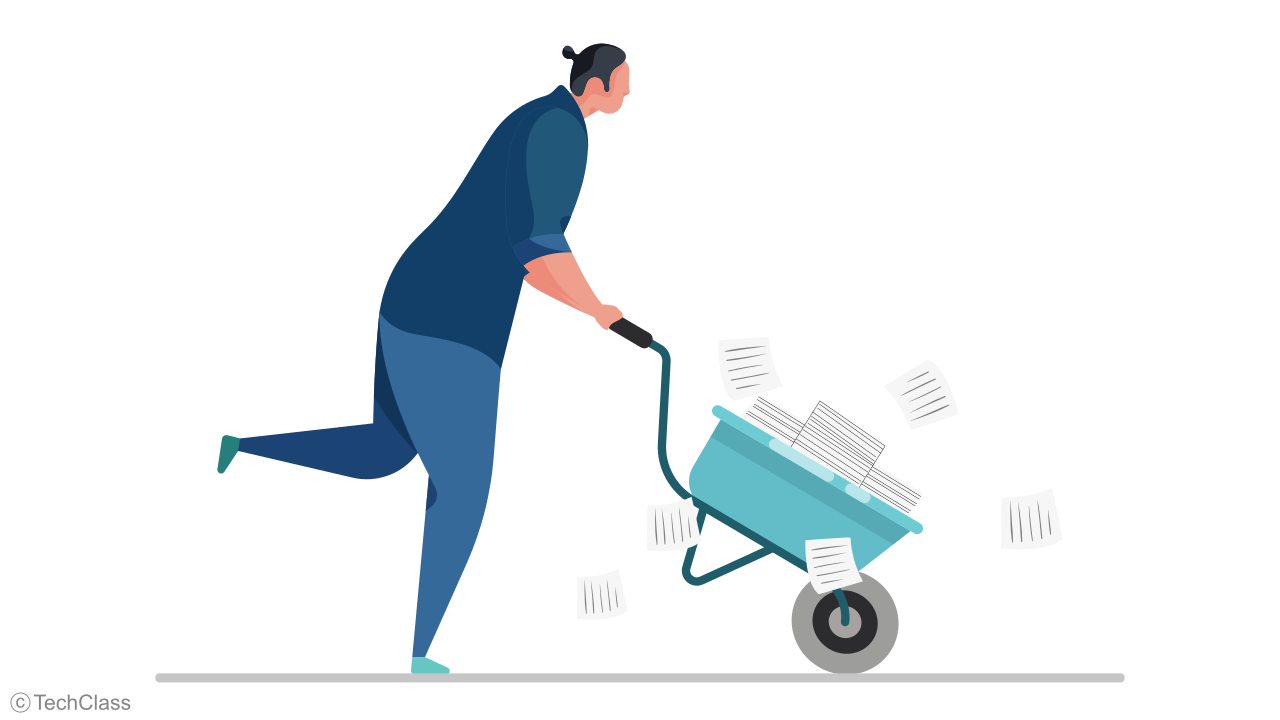
Collaborating with other teams
Unlike some routine job positions, data scientists don't work in an isolated environment to do their technical tasks. On the one hand, data scientists need to collaborate with different technical teams, such as data engineers, considering that each team has its priorities, and pipelines may hinder a perfect collaboration. On the other hand, data scientists have to communicate with non-technical company members. It means they should deal with how to present their technical results so that stakeholders or even customers can benefit from their outputs; otherwise, their solutions will be useless.

Securing data
In today's age, as we move more and more data to the cloud, there's a growing concern: how do we keep our data safe? Just like storing valuables in a bank, when we store our digital information online, it can catch the eyes of thieves or, in this case, hackers.
Companies, big and small, store private and sensitive information online. This data can range from customer details to secret business plans. If the wrong person gets their hands on it, it can cause much harm.
But here's the good news: data scientists have a toolkit to fight back. They can use artificial intelligence (AI) methods to up the security game. Think of AI as a digital security guard. It can spot unusual activities, detect potential threats, and even predict where future attacks might come from. By constantly learning and adapting, AI can help ensure our data remains locked away from those who shouldn't see it. For detailed insights and an immersive learning experience about data scientists and their role, be a part of our Introduction to Python for Data Science Online Training.
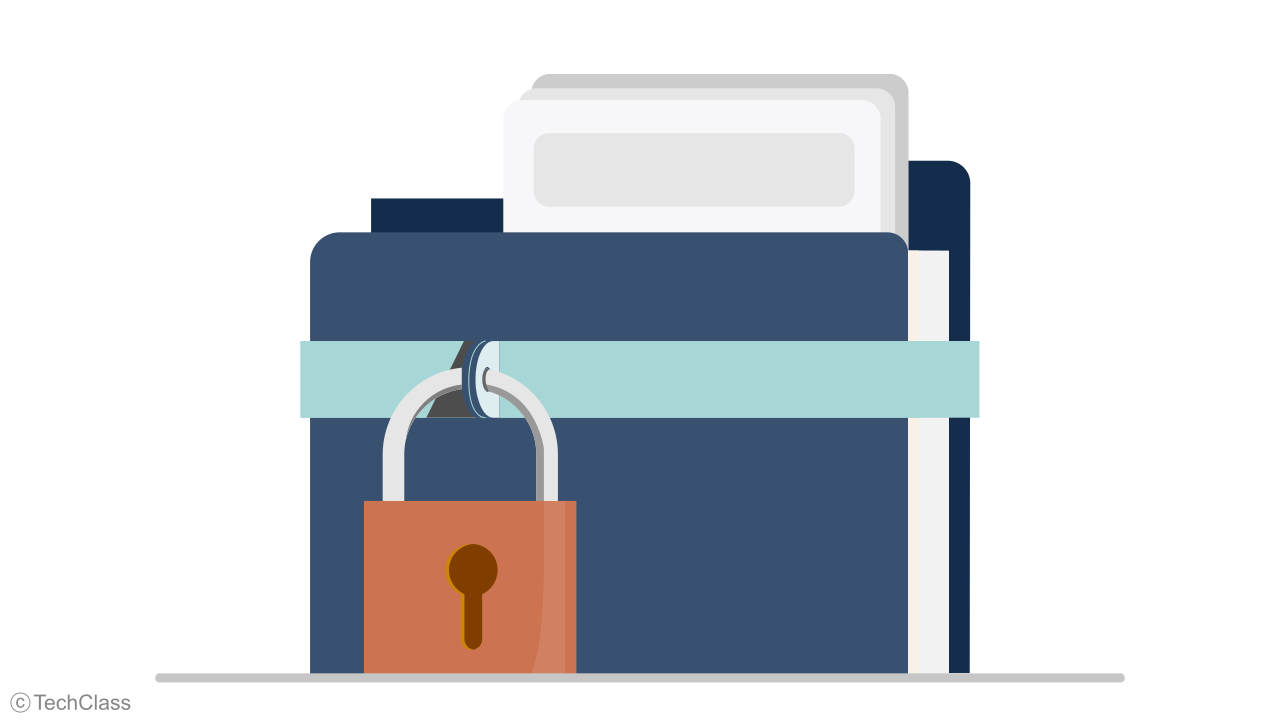